Congrats on Carolyn’s best poster award at Asilomar Bioelectronics Symposium! She also gave an oral presentation on her project “Wireless, Dry-Electrode Ear EEG for Drowsiness Monitoring,” recently published in Nature Communications. Well deserved!
Paper title: Wireless ear EEG to monitor drowsiness
Abstract: Neural wearables can enable life-saving drowsiness and health monitoring for pilots and drivers. While existing in-cabin sensors may provide alerts, wearables can enable monitoring across more environments. Current neural wearables are promising but most require wet-electrodes and bulky electronics. This work showcases in-ear, dry-electrode earpieces used to monitor drowsiness with compact hardware. The employed system integrates additive-manufacturing for dry, user-generic earpieces, existing wireless electronics, and offline classification algorithms. Thirty-five hours of electrophysiological data were recorded across nine subjects performing drowsiness-inducing tasks. Three classifier models were trained with user-specific, leave-one-trial-out, and leave-one-user-out splits. The support-vector-machine classifier achieved an accuracy of 93.2% while evaluating users it has seen before and 93.3% when evaluating a never-before-seen user. These results demonstrate wireless, dry, user-generic earpieces used to classify drowsiness with comparable accuracies to existing state-of-the-art, wet electrode in-ear and scalp systems. Further, this work illustrates the feasibility of population-trained classification in future electrophysiological applications.
Publication:
-
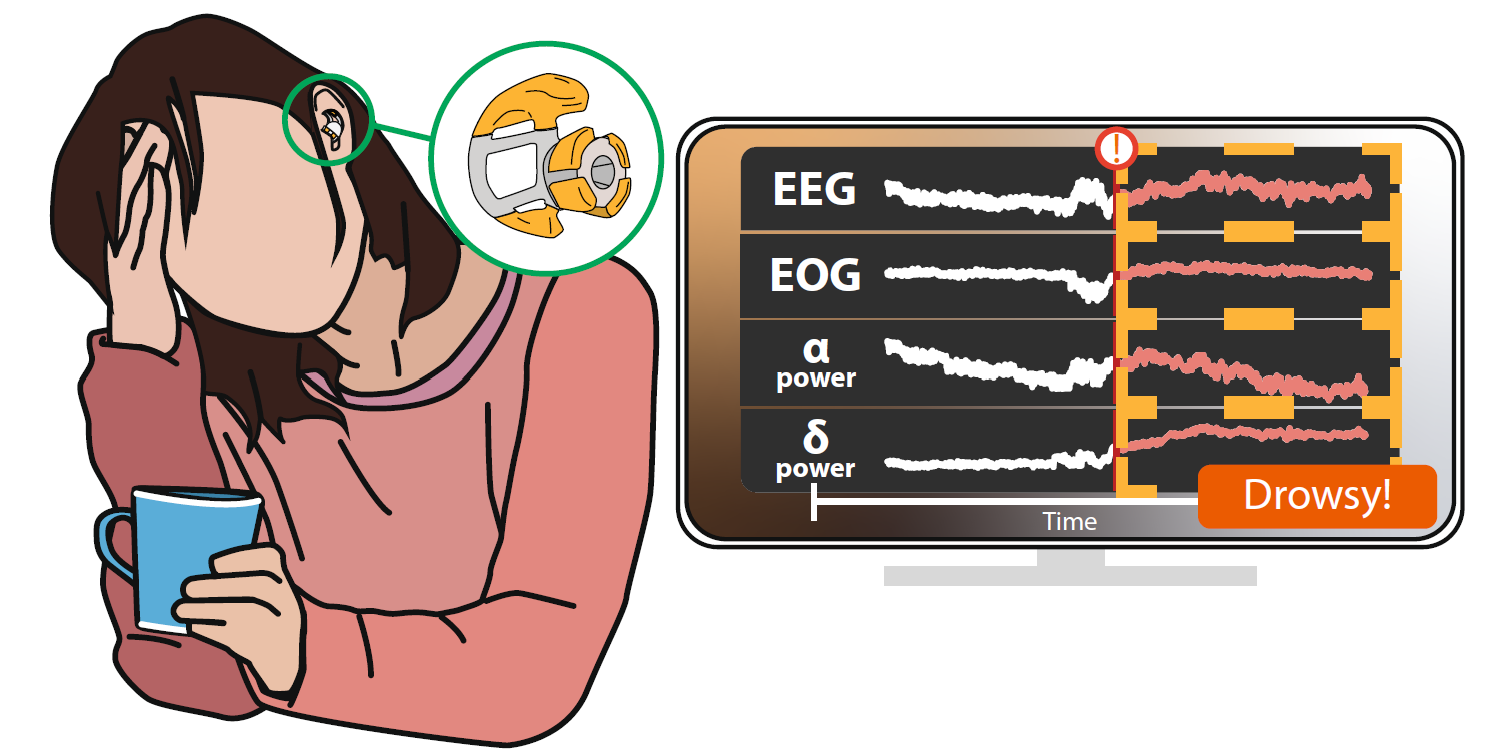
Wireless ear EEG to monitor drowsiness
Ryan Kaveh,
Carolyn Schwendeman,
Leslie Pu,
Ana C. Arias,
and
Rikky Muller
Nature Communications,
2024
15,
.
[Abstract]
[Bibtex]
[PDF]
Neural wearables can enable life-saving drowsiness and health monitoring for pilots and drivers. While existing in-cabin sensors may provide alerts, wearables can enable monitoring across more environments. Current neural wearables are promising but most require wet-electrodes and bulky electronics. This work showcases in-ear, dry-electrode earpieces used to monitor drowsiness with compact hardware. The employed system integrates additive-manufacturing for dry, user-generic earpieces, existing wireless electronics, and offline classification algorithms. Thirty-five hours of electrophysiological data were recorded across nine subjects performing drowsiness-inducing tasks. Three classifier models were trained with user-specific, leave-one-trial-out, and leave-one-user-out splits. The support-vector-machine classifier achieved an accuracy of 93.2% while evaluating users it has seen before and 93.3% when evaluating a never-before-seen user. These results demonstrate wireless, dry, user-generic earpieces used to classify drowsiness with comparable accuracies to existing state-of-the-art, wet electrode in-ear and scalp systems. Further, this work illustrates the feasibility of population-trained classification in future electrophysiological applications.
@article{Carolyn_drowsiness,
author = {Kaveh, Ryan and Schwendeman, Carolyn and Pu, Leslie and Arias, Ana C. and Muller, Rikky},
title = {Wireless ear EEG to monitor drowsiness},
year = {2024},
doi = {10.1038/s41467-024-48682-7},
publisher = {},
url = {https://doi.org/10.1038/s41467-024-48682-7},
journal = {Nature Communications},
volume = {15},
number = {},
thumbnail = {carolyn2024naturecomm.png},
pdf = {carolyn2024naturecomm.pdf}
}